TABLE OF CONTENTS
What is Reverse ETL? Here's everything you need to know in 2024
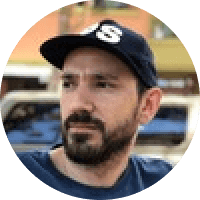
Syl is the Head of Growth & Operations at Census. He's a revenue leader and mentor with a decade of experience building go-to-market strategies for developer tools. San Francisco, California, United States
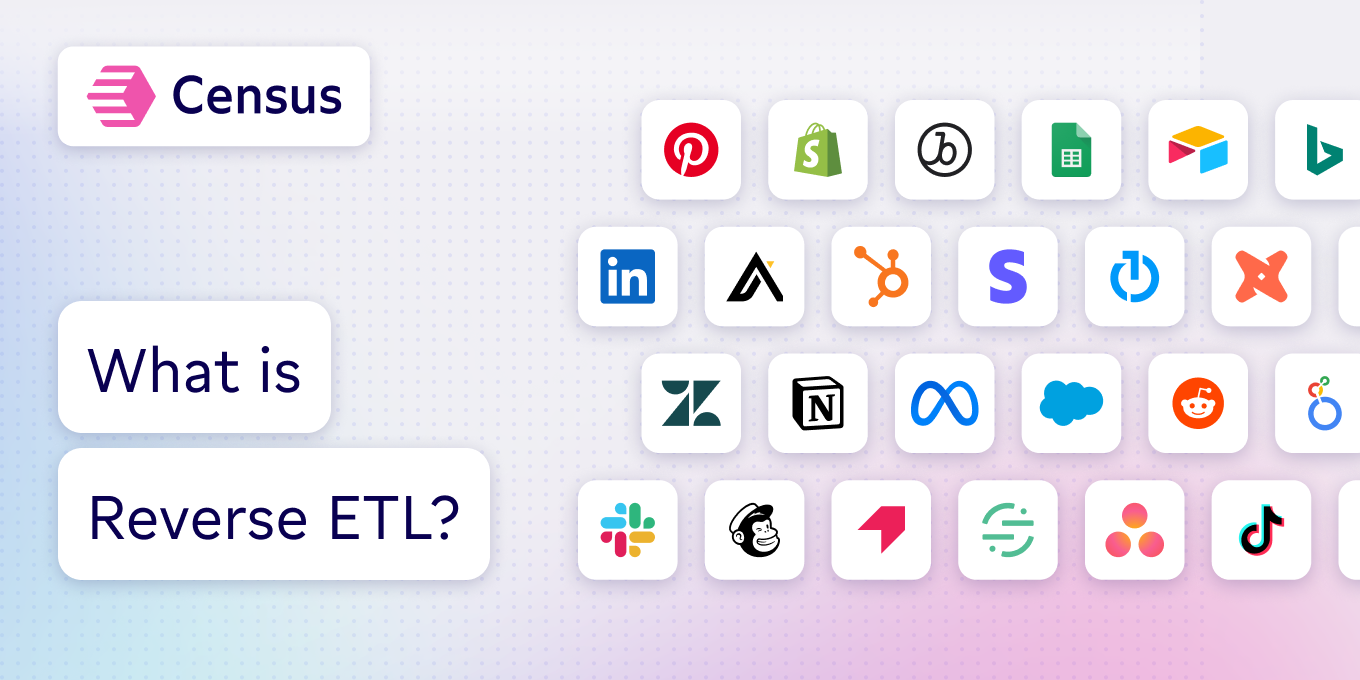
Do companies that care about their users dream of seamless data stacks? Absolutely.
For business folks, that looks like having up-to-date, actionable data right in their main tools (like Salesforce, Braze, and Marketo). For data teams, on the other hand, it's seeing the data they've improved with tools like Fivetran, Snowplow, and dbt being used to make every part of the business better.
In the past, data stacks haven't fully achieved these dreams—but they have come pretty darn close.
Using tools like Fivetran, data teams gather and organize data from mobile and web apps into one main data place, known as a data warehouse or data lake. At this point, they have the ability to examine the data closely, create advanced prediction and machine learning models, and supply information to business teams to help them make decisions.
But, even then, there's still a missing piece—what we call "the last mile"—between the warehouse and the tools the business workers use.
The solution to this "last mile" problem—taking you from your current data situation to your dream data scenario—is a little something called Reverse ETL. Technically speaking, Reverse ETL is the process of syncing data from a source of truth like a data warehouse or data lake to a system of actions like CRM, advertising platform, or other SaaS app to activate data.
In simpler terms, though, it's the difference between making decisions based on your data and finally being able to take action to realize your data dreams.
Let’s dive in.
What is reverse ETL?
We'll say it again for the folks in the back:
Reverse ETL is the process of syncing data from a source of truth like a data warehouse or data lake to a system of actions like CRM, advertising platform, or other SaaS app to activate data.
That’s basically just a fancy way of saying reverse ETL lets you move data about your users from your central storage repository and makes it available for frontline business teams to use in their favorite tools.
However, to really understand the power of reverse ETL (and why it’s not just another data pipeline), we first need to take a quick look at what traditional ETL pipelines made possible for business and data teams.
What’s in a name: ETL vs reverse ETL
The traditional extract, transform, load (ETL) data pipeline has remained largely unchanged since the 1970s: extract the data from the source, convert it to a usable format (or transformation), then load it into your data warehouse or lake.
The advent of flexible data pipeline tools like Fivetran has also made it possible to load your data into the warehouse or lake and then use your storage target to transform it (referred to as ELT). These ETL/ELT enabled companies to combine data from multiple sources into a single source of truth to inform business intelligence decisions.
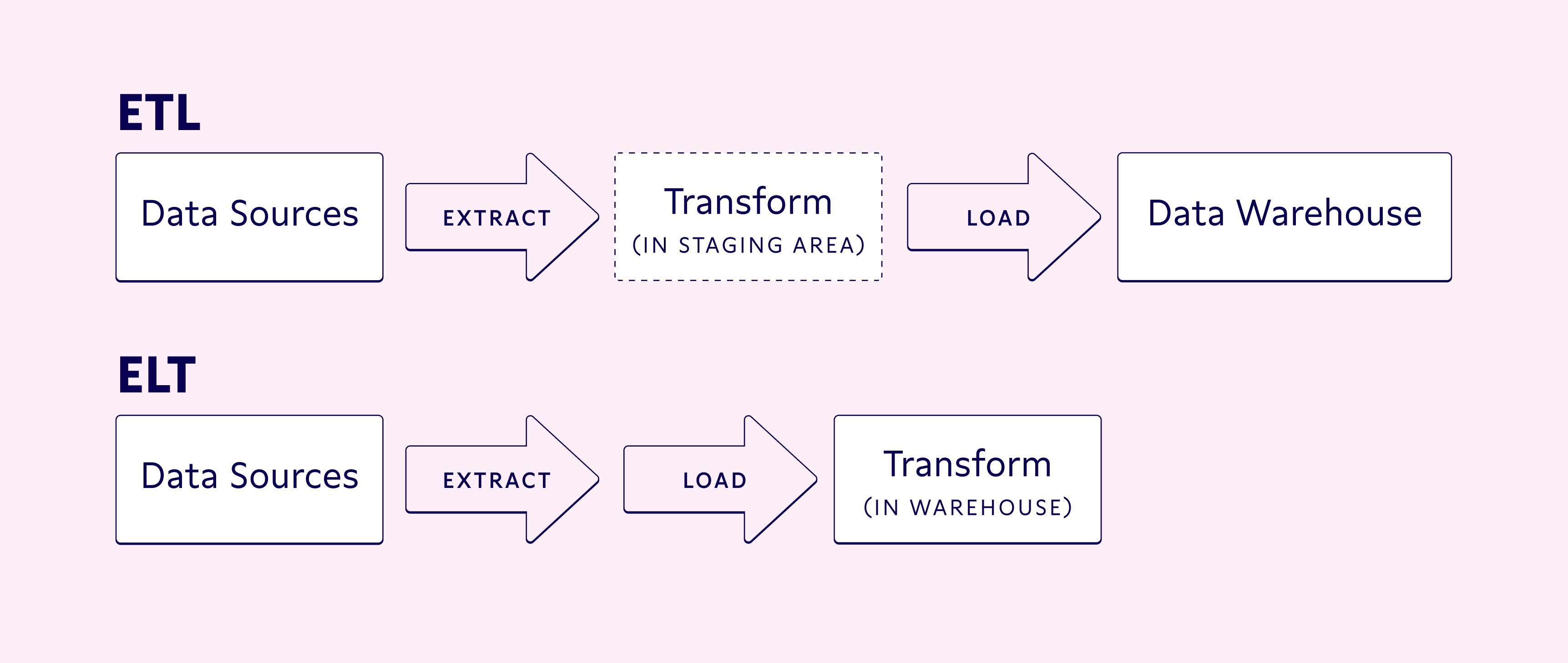
This version of the modern data stack worked well when data sources were more limited (i.e. there was less data volume) and the data engineers who supported these stacks had ample bandwidth to process and answer questions about data. As you’ve probably experienced, that’s no longer the case and teams need more sophisticated tools to achieve the dream of data activation.
This reverse journey à la reverse ETL makes operational analytics possible. Reverse ETL tools flip the Fivetran role, extracting data from the warehouse, transforming it so it plays nice with the target destination’s API (whether Salesforce, HubSpot, Marketo, Zendesk, or others), and loading it into the desired target app.
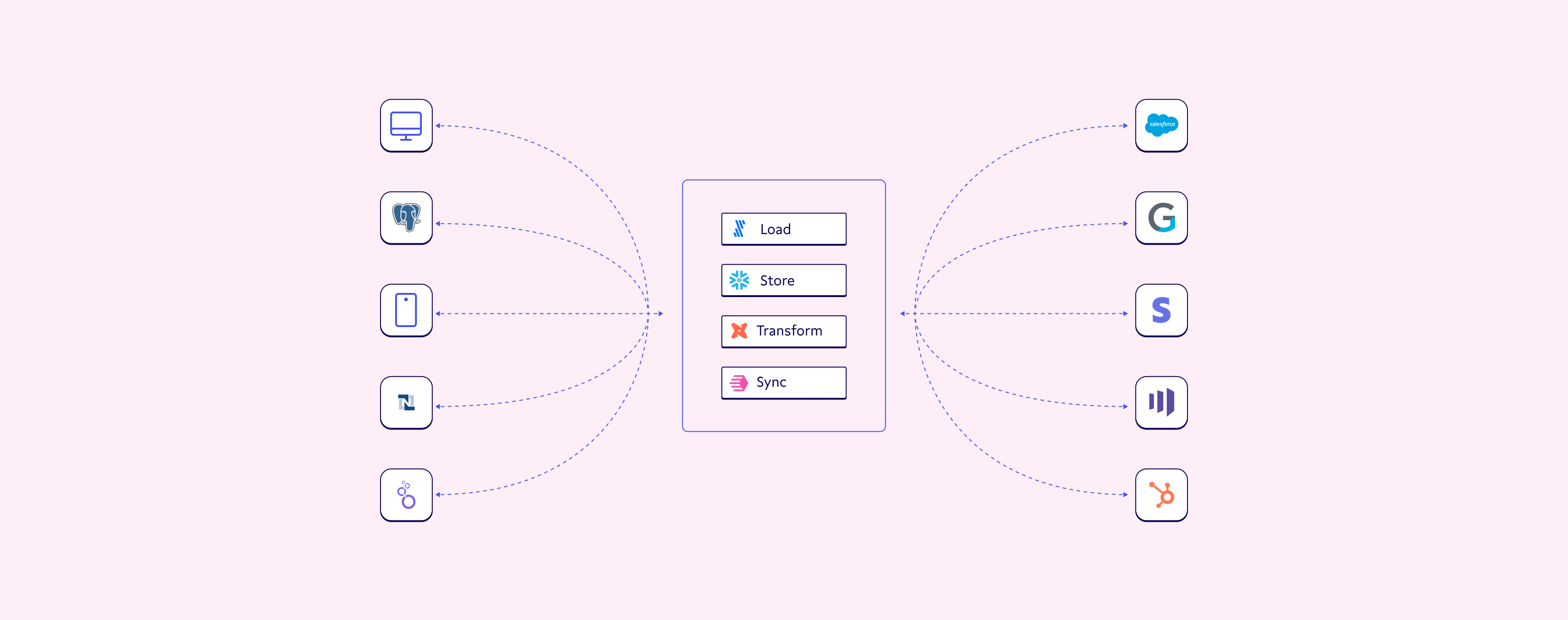
Modern data stack 2.0: The era of operational analytics
The reverse ETL-inclusive modern data stack is the modern data stack 2.0. The growth in popularity of this new generation of data stack is emblematic of an important trend: Companies need to move data capabilities out of centralized silos and embed them within teams across business functions.
Related reading: Why reverse ETL (and why reverse ETL now)?
Reverse ETL equips these teams with detailed data inside the tools they're already using like Salesforce or HubSpot, empowering them to be more effective in their day-to-day work. The reverse ETL process effectively aligns your organization and applications around your source of truth. From there, business teams can build a shared, deep understanding of customers like never before.
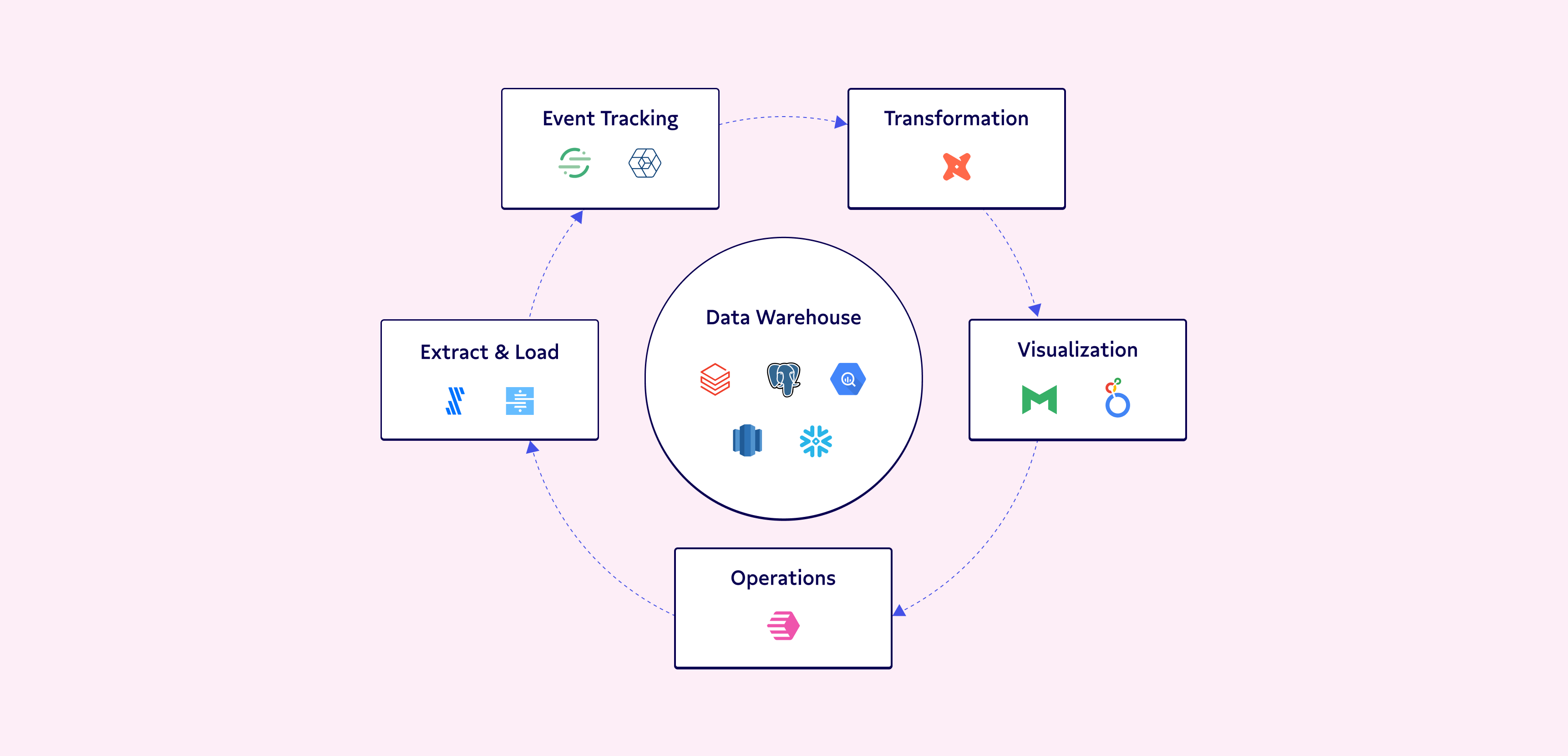
The continuous flow of data--from raw data being pulled into apps to data being modeled to data being deployed into each app--creates a virtuous loop of operational analytics. And it’s only possible with reverse ETL.
This new genre of data tools closes the feedback loop that separated DataOps from DevOps and makes it possible for teams to deploy relatively real-time data and insights to core apps and services. - Boris Jabes, CEO at Census, The Operational Analytics Loop: From Raw Data to Models to Apps, and Back Again
The modern data stack 2.0 generally consists of the following tools performing four key functions to close the operational analytics loop:
- Data integration: Also referred to as collection, this is an ETL tool like Fivetran or Snowplow that integrates your data sources into your warehouse.
- Data storage: A data warehouse that can store structured and unstructured data in one place like Google BigQuery, Snowflake, or Amazon Redshift.
- Data modeling: A modeling tool like dbt comes pre-configured with a massive library of data models to make your data usable in different situations.
- Data activation: A reverse ETL tool like Census will pull data out of your warehouse, validate it, and load it into applications that need it like Salesforce or Zendesk.
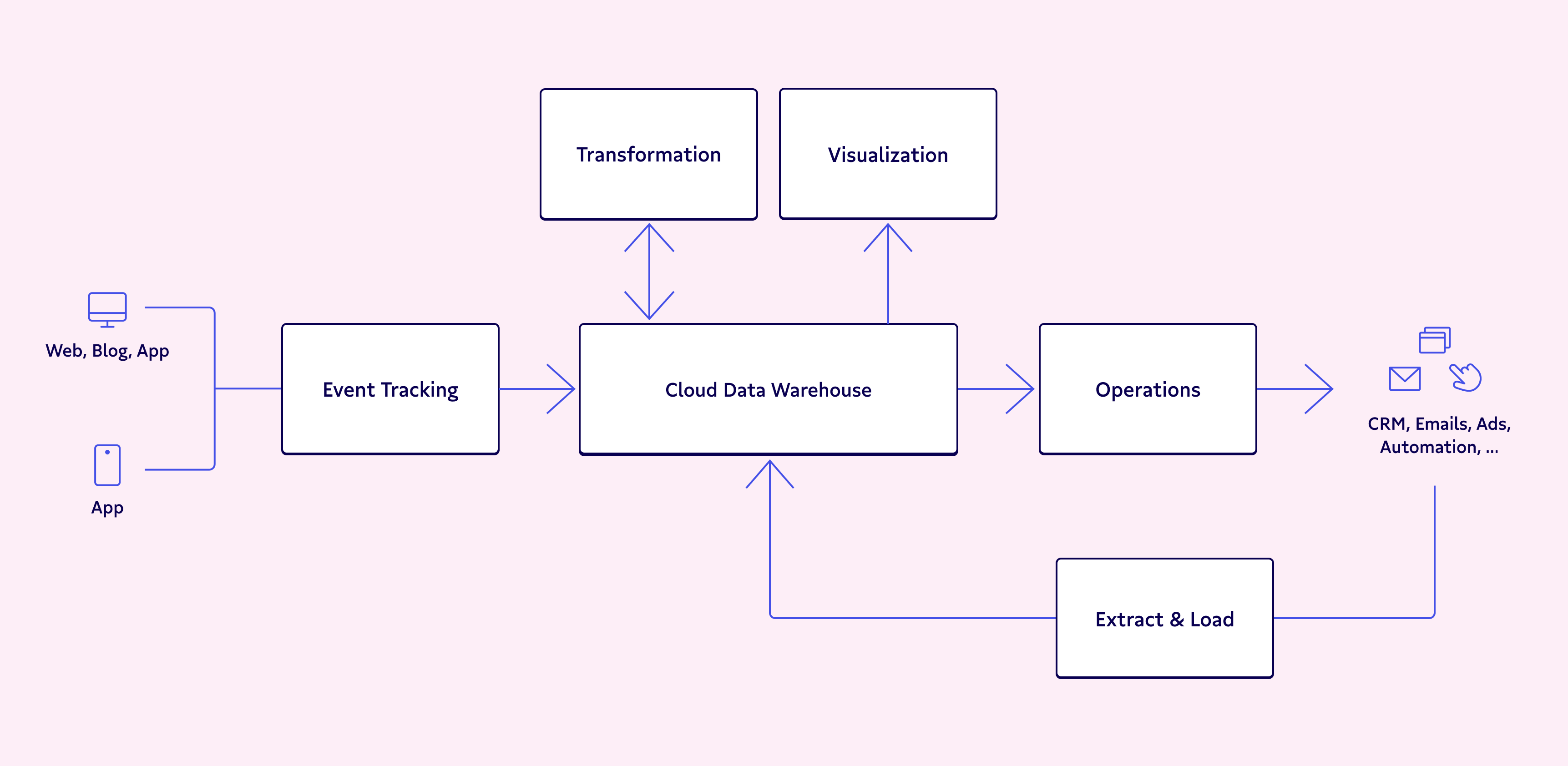
As more teams within an organization require data to drive their daily operations, reverse ETL will become necessary to support democratizing data at scale.
Why you need reverse ETL
Without a reverse ETL tool, your data, and the insights from it, are locked within your BI tools and dashboards. This won’t fly in the era of product-led growth, which pushes companies across the B2B and B2C spectrum to improve customer experiences with personal, data-informed strategies.
As we touched on above, the key to this personal customer connection lies in operationalizing our data. Before reverse ETL, data pipelines were built for analytics alone (which meant data efforts were primarily focused on understanding past behavior). Now, companies can architect their data stacks to fuel future action, as well as understand past events (aka operational analytics).
At its core, operational analytics is about putting an organization’s data to work so everyone can make smart decisions about your business. - Boris Jabes, Census CEO
Reverse ETL lives at the heart of operational analytics at scale, constantly pumping real-time customer data into third-party applications to ensure when it comes time to make a decision, the right person has the right data to do it.
When teams across an organization work with synced data, traditionally difficult to automate tasks become much more straightforward. For example, reverse ETL makes it possible to intervene in the customer journey at just the right time by connecting your CRM and email platform to your data warehouse. This means more successful outreach campaigns and more delighted customers.
Reverse ETL use cases
Connecting teams throughout your organization to the warehouse using reverse ETL empowers them with data enriched with valuable context about what your customers are doing in real-time. As we discussed, an operational analytics approach puts data into the hands of people to inform their day-to-day operations. Let’s look at some leading use cases of how customer success, sales, marketing, and data teams benefit from reverse ETL.
CS success with better, faster data and reverse ETL
Customer success teams are responsible for more business outcomes than ever before, from traditional support efforts to product adoption to retention efforts to expansion initiatives. To meaningfully contribute goals in each lane of their job descriptions, customer success teams need high-quality, trustworthy data when they need it in the tools they rely on.
Industry-leading companies like Loom, Atrium, and Bold Penguin have upgraded their modern data stacks with reverse ETL to accomplish some awe-inspiring milestones, including but not limited to:
- Replacing hard-to-use DIY reverse ETL or P2P solutions.
- Helping customer success and sales better collaborate to reiterate product value at just the right time to customers.
- Making account type and hierarchical ticket prioritization a reality.
- Supporting self-serve data capabilities for the customer success team, decreasing their reliance on the data team.
- Reducing response times for common support issues from days to minutes.
As we've said before, reverse ETL isn't about adding another tool to your stack, it's about empowering people with better data so they're unblocked to do their best work. With reverse ETL, customer success and ops teams can quickly and easily tap into powerful data insights to better serve customers and contribute to growth goals.
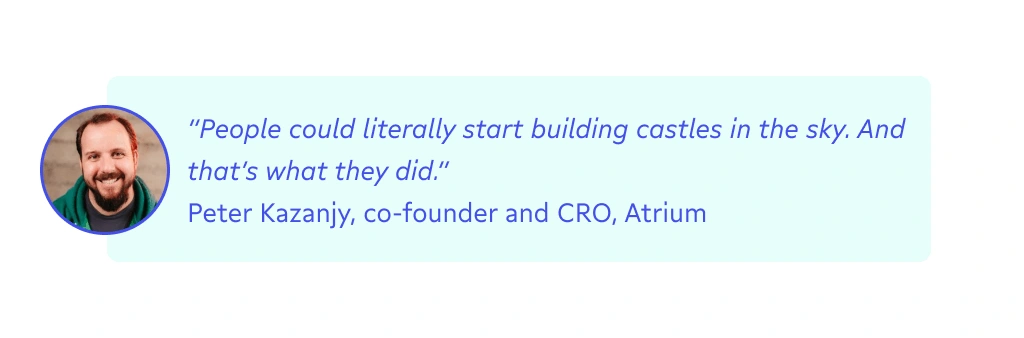
Need some CS inspiration? Check out our customer service use-cases in our Good to great series highlighting the best and brightest in CS.
Sales team heroics: Up-leveling sales with reverse ETL
In the era of product-led growth, it's no longer enough to just have a great product, you need to foster a good relationship with every lead from the start.
Often, reverse ETL is the difference between a missed connection and a life-long customer bond.
Here are some of the people-first use cases cutting-edge sales teams at companies like Figma, LogDNA, and Snowplow Analytics have unlocked using reverse ETL:
- Improved understanding of what features customers loved most and where each customer was in their life cycle.
- Unified understanding of customers and the organizations they belonged to with identity resolution.
- Improved AE and AM focus and effectiveness with lead scoring.
- Real-time sales forecasting in Google Sheets.
- Gave the sales team access to high-quality behavioral data in the tools they loved to help them meaningfully connect with prospects (without engineering favors).
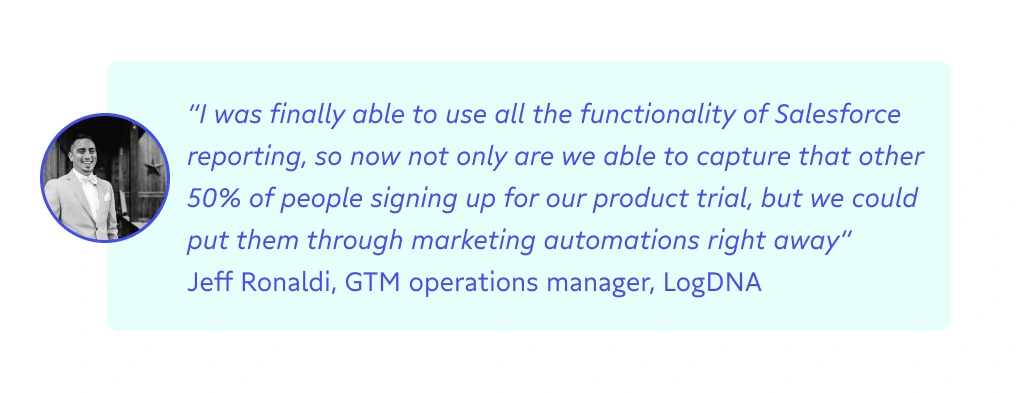
Need some sales inspiration? Check out sales use-cases in our Good to great series highlighting the best and brightest in sales.
Building better, faster, stronger marketing teams with reverse ETL
With customers' expectations climbing higher every year, it's more important than ever that marketing teams have access to complete, fresh data to attract and convert new customers (and delight current users).
Industry-leading marketing teams--like the ones found at Notion and Canva--have cracked the code on data-driven marketing operations with reverse ETL. Here are a few examples of what you too could do with reverse ETL:
- Eliminate the need for custom integration requests and manual email address uploads.
- Quickly and easily get data into Salesforce workflows for lead scoring and PQLs.
- Get the full functionality out of all your existing tools (and unlock tools on your marketing team's wishlist).
- Leverage more actionable user data to drive segmentation and personalization.
- Fuel faster experimentation with ad targeting and user propensity scoring.
With reverse ETL marketing teams can build hyper-personalized marketing campaigns by merging product, support, and sales data to power customer segmentation. No more missed opportunities.
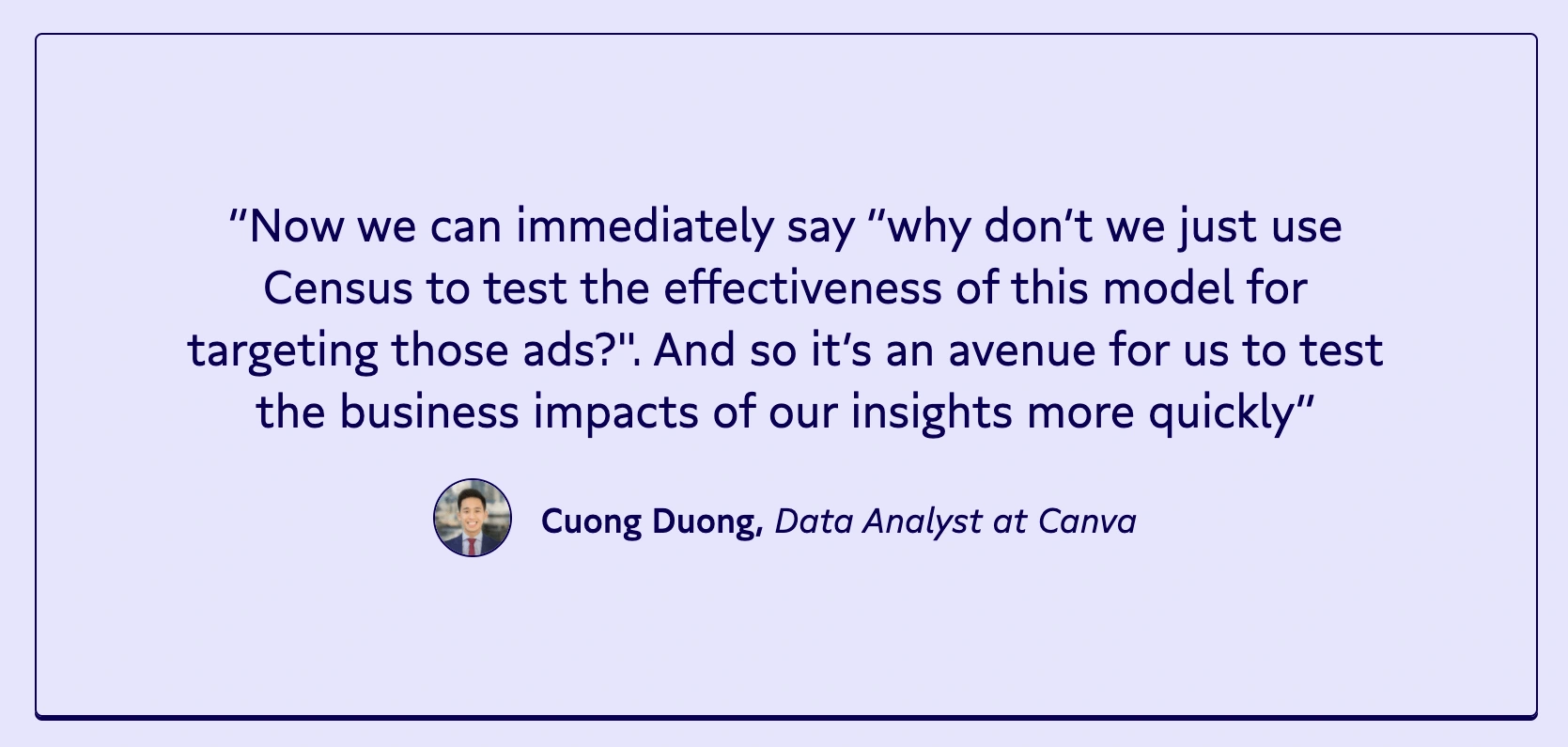
Need some marketing inspiration? Check out marketing use-cases in our Good to great series highlighting the best and brightest in marketing.
Reverse ETL helps data teams step into their power
No one got promoted for building ETL/reverse ETL. When data teams spend their team building and maintaining bespoke integration solutions they're blocked from doing the innovative, high-impact data work they were hired for.
With reverse ETL, data teams at companies like Canva, Clearbit, and Loom have been able to not just better meet the ad hoc needs of business teams, but carve out time to change the role and culture of data entirely at their organizations. This kind of visionary data work is what nearly every industry in the game needs to embrace to move into the future.
Here are some examples of what reverse ETL can do for data teams:
- Reduce the time data teams spend doing tedious integration build work and more time doing exciting, engaging data work.
- Increase the ability of data leaders to advocate for the skill sets of the data team and establish data team as a key stakeholder.
- Foster happier internal customers of data, which means more people take strategic action from data.
- Generate fresher, more accurate data for outreach campaigns.
- Give the data team complete control of the data flow from ETL to the frontline tools.
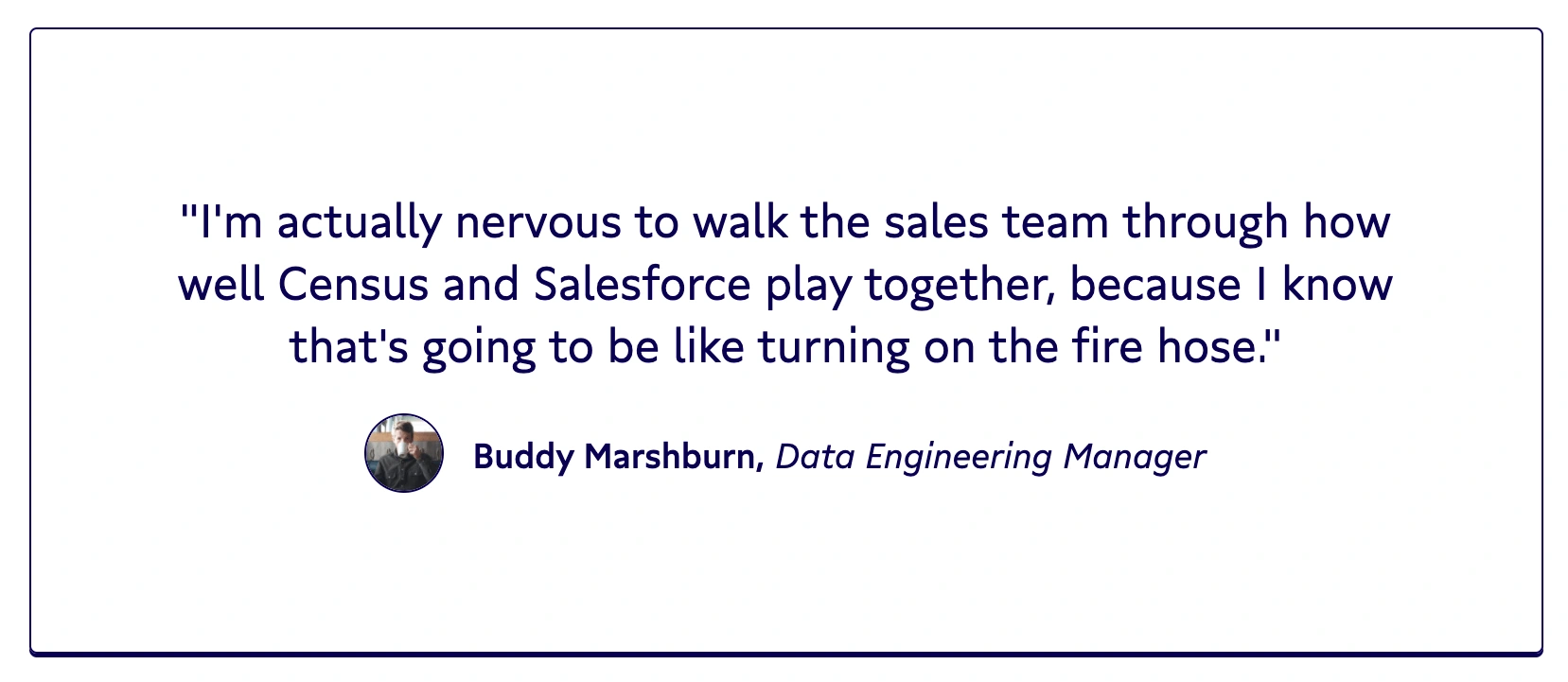
Need some data team inspiration? Check out data-team success stories in our Good to great series highlighting the best and brightest in data.
Reverse ETL vs point-to-point integrations
The no-code, plug-and-play nature of a point-to-point platform like Workato, Zapier, or Mulesoft often entices teams without dedicated technical or data resources to set up any necessary integrations. But relying too heavily on these quick fixes can quickly get messy as your data stack grows.
Fully integrating point-to-point solutions with your data stack requires exponentially more connections as your stack grows. The number of connections grows by the square of the number of applications, meaning eight apps could require as many as 64 distinct connections to keep your entire stack in sync.
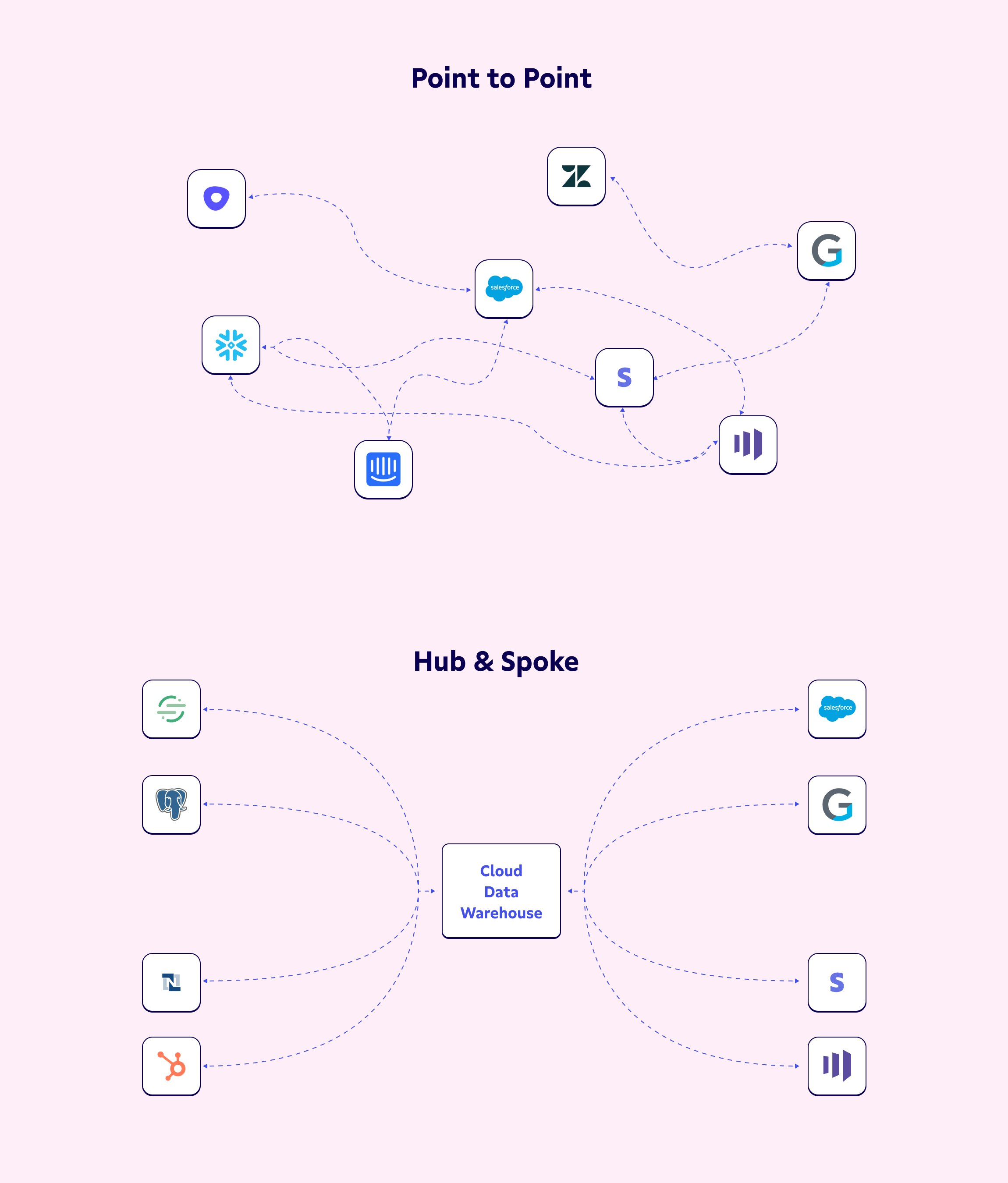
Things can get messy quickly when you’re trying to manage too many integrations.
But with all of the customer data you already have sitting in your warehouse, there’s a better way. Instead of a messy, spaghetti pile of point-to-point integrations, you can use reverse ETL to architect your data infrastructure as a series of orderly spokes around a central hub (data warehouse). This creates a single source of truth informing each application and workflow within your stack to make you truly data-informed.
What to look for in a reverse ETL tool
As is the case with most software, when looking for a reverse ETL tool you’ll have to decide whether to buy an established product or attempt to build a bespoke solution with your resources on hand.
Building a custom reverse ETL pipeline may seem attractive, but it comes with the added complexity of not only engineering each individual connector but maintaining them against ever-changing destination APIs.
If you want to save your business teams from endless ticket filing (and save your engineers from having to address all those tickets), it’s time to consider a managed reverse ETL solution from an expert vendor. Here is a high-level overview of the seven key features to look for in a potential reverse ETL tool:
- Connector quality: A reverse ETL tool is only as useful as the applications it connects to. Look for the connections you need today and the specific features of each.
- Sync robustness: Syncing is arguably the most important feature and should be fast, be reliable, sync only data that’s changed, and be automatable.
- Observability: Your reverse ETL should offer alerting, integrations with monitoring tools, detailed logs, and the ability to rollback syncs, if necessary.
- Security and regulatory compliance: Vendors should have security credentials like SOC Type I or II, encrypt data in transit and at rest, and use best-in-class security for APIs.
- SQL fluency and ease of use: To be as user-friendly as possible, your reverse ETL tool should be SQL friendly, allow for easy modeling, and have an intuitive user interface.
- Community and vendor support: Make sure your reverse ETL vendor has a high commitment to SLAs, readily available support and in-app support, and good documentation.
- Transparent pricing: When buying a reverse ETL tool, make sure you know if the vendor charges by consumption, number of connectors, or fields per sync.
If you do your due diligence when selecting reverse ETL vendors, you’ll have the ultimate tool in your toolbox to ensure you get the most of your data today and as you scale in the future.
Reverse ETL makes your data (and the teams that use it) more efficient
When front-line teams can self-serve highly detailed customer data, translated, validated, and formatted for their favorite tools, data teams can spend less time crunching numbers and running reports and more time using their insights to inform business strategy.
The traditional role of data or analytics teams was, first and foremost, to report on how a product or campaign performs over time and serve the requests of the business teams they support.
This type of reporting and support was useful for monitoring the long-term health of your user base or high-level budget planning, but it couldn’t power automation or help customer success managers triage incoming support requests.
Today, data teams have embraced a whole new set of sophisticated analytics engineering skills. Unblock them and let them use these skills (you’ll be amazed at what they can do, we promise).
With reverse ETL in place, modern data teams turn data warehouses into the central nervous system of an organization, fueling email marketing, customer support tools, sales tools, or even financial models. This means more successful business teams that can self-serve deep, useful data and more efficient DataOps overall.
Want to see how a reverse ETL system can change your day-to-day work and, your whole world of data, from day one? Schedule a demo with Census and we’ll show you what we can do. Or start checking it out for free today! ✨
Frequently asked questions
Why is reverse ETL important?
Reverse ETL is crucial in today's data ecosystems because it makes it simple for businesses to transfer data from their data warehouses to other systems. In other words, they can activate the data they have gathered to provide better customer experiences, improve internal processes, and more. See all the reverse ETL use cases.
Why is reverse ETL taking off?
Reverse ETL is taking off because it's increasingly important for companies to be able to access their data in real time and ideally as soon as it's generated. Reverse ETL enables them to extract data from their data warehouses and make it available to other systems at the speed companies have come to expect today.
Is reverse ETL the same as ELT?
Reverse ETL and ELT are different processes. ELT involves extracting data from source systems, loading it into a target system, and then transforming it within that system. Reverse ETL, on the other hand, involves extracting data from a target system, transforming it, and making it available to other operational systems.
While both processes involve data transformation, they differ in their purpose and direction of data flow. ELT is used primarily for data warehousing and business intelligence purposes, while reverse ETL is used for operational purposes such as syncing data with customer-facing applications or partner systems.